Introduction Data Analytics Tools
In the contemporary landscape of data-driven operations, businesses hinge on their strategies and decisions based on the insights gleaned from data analytics. Aspiring data analysts and seasoned professionals alike must grasp a diverse array of data analytics tools to navigate this terrain effectively. In this article, we’ll delve into 10 indispensable data analytics tools crucial for informed decision-making and strategic initiatives. Whether you’re venturing into the field as a novice or aiming to broaden your expertise as a seasoned data analyst, these tools serve as pillars of success in the realm of data analytics. Even if you are enrolling in a Data analyst course online, you must ensure that these topics are discussed in sufficient detail in the course.
1. SQL (Structured Query Language)
SQL (Structured Query Language) is a basic instrument for information investigation with a consistent connection point to cooperate with social data sets. A decent information on SQL is vital in any information expert’s life since it makes them ready to handily remove, make due, and control information. SQL dominance empowers individuals to work with complex questions so they can see what is inside the huge arrangements of information regardless of the kind of data, either marketing projections, client subtleties, or even monetary records.Using SQL, analysts can navigate through the complexity of database structures, retrieving only a specific subset of data and performing aggregation or transformation as required. Across various industries and functions within an organization, such as marketing & sales, finance, and operations, among others. This versatility has made it an essential tool. Professionals trained in SQL have a skill set that allows them to make sense of diverse datasets, hence enhancing decision-making in this era driven by big data analytics, which eventually leads to organizational success. Whether working on structured and traditional relational databases or new formats of storing data, SQL remains foundational to the effective analysis of data.
2. Excel
Excel still stands out as one indispensable program used in handling and managing raw business statistics courtesy of its ease-of-use coupled with a rich feature set, among others. It also applies to various tasks related to statistical analysis, such as basic manipulation and visualization, as well as complex model building and analysis. Depending on how much you know about analyzing information using computers, you will find Excel friendly for different analytical needs.
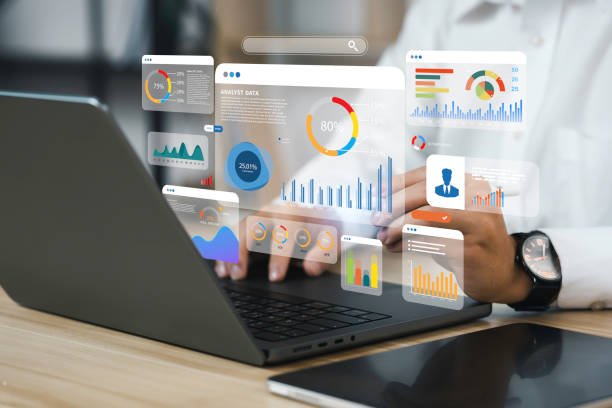
With its familiar interface and wide range of features, excel caters to all levels; be it mere beginners or experts, when it comes to organizing, manipulating, or presenting data no other software does it better than this application program is herein referred to as Excel. Built-in functions include pivot tables, charts, and formulas, which aid in quick insight development through efficient processing plus visualization, without which it would be impossible. Furthermore, excel has a good compatibility especially with other data analysis tools as well as platforms that have been embraced by this new era of using “Big Data” workflow.
3. Python
The simplicity, flexibility, and rich library ecosystem are some of the reasons why Python emerged as a favorite programming language for data analysts. Such libraries like Pandas, Numpy, or Matplotlib have made it possible to perform numerous tasks such as data manipulation, statistical analysis, machine learning, and data visualization, among others. For instance, whenever raw information needs to be cleaned before performing advanced predictive modeling or when creating interactive plots that can catch people’s attention visually, a strong language like Python is quite handy.
Python is easy to learn due to its syntax and documentation system, which makes it accessible even to non-programmers. Additionally, seamless integration with other tools/processes for analyzing data further increases its popularity among users of all levels who use it for different analytical tasks related to data processing. As organizations increasingly rely on data-driven insights to drive decision-making processes, Python’s role in facilitating efficient and effective data analysis continues to expand, solidifying its position as a cornerstone tool in the data analyst’s toolkit.
4. R
R has gained a lot of prominence lately as an extensively used programming language for statistical computing and data analysis purposes. This includes a large number of packages available within R, including those dealing with various aspects of analytics, hence providing a wide selection range applicable in diverse situations by these professionals. Some examples include exploratory data analysis (EDA), hypothesis testing (HT), and regression analyses (RA). In many cases involved in activities such as cleaning up datasets prior to running models based on statistics through generation quality visualizations for publishing purposes amongst others would require one skilled in software like R, particularly statisticians and actuaries alike need this assistant
5. TensorFlow
TensorFlow is an open source software library conceived by Google Brain for conducting machine intelligence tasks such as neural networks and deep learning without any hindrance. It provides both high-level APIs for constructing deep learning models as well as lower-level interfaces used to build and deploy customized architectures from scratch if necessary. Starting from Android smartphones and iPhones to Raspberry Pi single-board computers or huge clusters of GPUs in the cloud – TensorFlow can run on almost any recent computing device.
To enable developers to easily create scalable solutions leveraging artificial intelligence capabilities regardless of their proficiency level in AI programming, Google has released TensorFlow Lite, which is a lightweight version of its popular AI development framework specifically optimized for mobile devices and small embedded systems. This model execution platform supports various hardware accelerators extensively utilized in Android smartphones powered by Qualcomm Snapdragon chips.
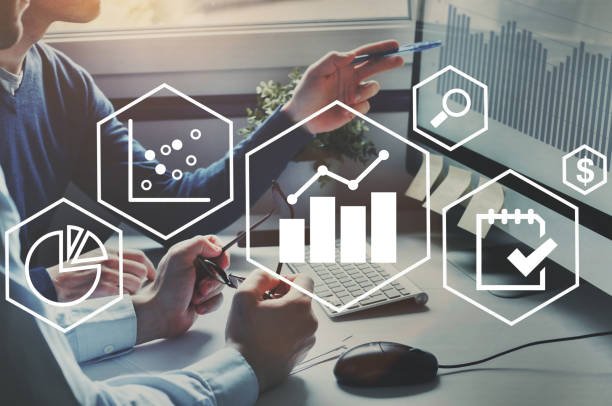
6. Microsoft Azure Machine Learning Studio
In this case study we present a detailed description of how MS Azure ML studio was used by our team during the creation of an automated system that predicts future sales volumes based on historical demand patterns registered at different retail points located within the country. The good news is that this platform does not require any programming skills, so practically anyone can try using it. To do so, they should create an account on the official website of MS Azure ML Studio and start working with preloaded models or train their own ones from scratch.
Azure Machine Learning Studio by Microsoft is a web-based integrated development environment (IDE) that enables building and deploying machine learning models at scale. The Azure Machine Learning Workbench supports data scientists in testing and validating hypotheses by creating experiments using numerous data sets. A key component of this service is the ability to develop models in Python-based Jupyter Notebooks that leverage open-source libraries such as TensorFlow, PyTorch, or Keras.
7. Amazon SageMaker
It has been designed to aid in building, training, and deploying all kinds of machine learning models at high speed with minimal overhead regarding infrastructure management. AWS Sagemaker is a fully managed service used for constructing, training, and deploying machine learning algorithms based on user-defined mathematical formulas or algorithms developed by other companies including Google Corporation, Microsoft Inc., and IBM Corporation among others. With its declarative coding style, developers can quickly build complex deep-learning models without worrying about low-level details.
Amazon Sagemaker is a fully managed cloud platform specifically created to facilitate building, training, and deploying AI/ML models without requiring expertise in distributed systems engineering aspects like fault tolerance or distributed model caching mechanisms. This ecosystem comes complete with notebooks for interactive coding along with frameworks like MXNet, PyTorch, etc., so you don’t have to install anything locally anymore! Developers can create custom containers containing both their application code as well as runtime dependencies leveraging AWS Elastic Container Registry (ECR).
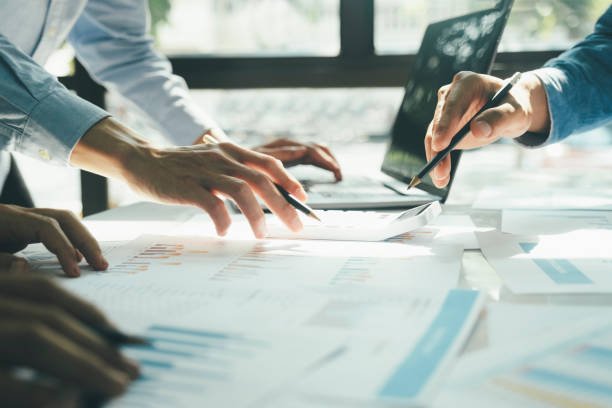
8. Apache Spark
Apache Flash is a quick and adaptable bunch registering structure particularly for enormous information investigation. It is popular for its in-memory handling ability that permits information researchers to run complex changes, AI calculations as well as stream handling with high effectiveness. Also, its help of various programming dialects and libraries builds the quantity of clients favoring it while permitting them to utilize their recognizable instruments.
Flash is a tough and elite presentation stage for information investigation, be it constant streaming or disconnected cluster handling of huge datasets. The appropriated design guarantees smooth parallelism on bunches of machines, working with quicker information handling at scale. Besides, adaptation to non-critical failure components and strength highlights incorporated into Flash make it more solid consequently appropriate for associations working under changing circumstances with enormous volumes of information.
9. Google Analytics
Google Investigation is a persuasive web analyzer that assists the following of site with dealing, client conduct and commitment measurements. With its strong detailing abilities and incorporation with other Google items, Google Investigation gives important bits of knowledge into site execution, crowd socioeconomics, and promoting adequacy. Whether you’re enhancing site content, estimating effort return on initial capital investment, or recognizing transformation open doors, Google Examination is an important device for information-driven dynamic in the computerized domain.
10. SAS (Statistical Analysis System)
SAS is a comprehensive software suite for advanced analytics, data management, and business intelligence. With its wide range of modules and capabilities, SAS enables data analysts to perform complex statistical analysis, predictive modeling, and data mining tasks. Whether you’re analyzing healthcare data, financial transactions, or customer demographics, SAS provides a robust and scalable platform for deriving insights and making informed decisions.
Conclusion
All in all, dominating these 10 fundamental information examination devices is pivotal for information experts looking to succeed in their field and drive powerful direction. From SQL and Succeed to Python, Scene, and then some, these devices give the establishment to separating, investigating, and imagining information to uncover bits of knowledge and drive business results. Whether you’re a fledgling hoping to break into the field or an accomplished proficient looking to extend your range of abilities, capability in these devices will engage you to prevail in the dynamic and information-driven universe of information examination.Embark on your journey today with data analyst courses that cover these essential tools and techniques, and take your data analysis skills to the next level.